With ChatGPT going viral, I figured it was time for my first post on AI.
2022 has been a massive year for AI. Yes, there have been incredible technology breakthroughs but that can take a backseat. I don't want to lose readers 3 sentences in with boring tech jargon. The year has been hard enough.
IMO, the most important AI breakthrough of 2022 has been getting society comfortable with using AI, trusting AI and having fun with it.
And Dalle-2 and ChatGPT have done just that —
Over the past 6 months, OpenAI built and released both Dalle-2 and ChatGPT. OpenAI is an AI research program founded by Elon Musk & Sam Altman.
Dalle-2 and ChatGPT have led to a huge influx of normal people using advanced AI, consuming advanced AI, sharing advanced AI. People love it.
Dalle-2 and ChatGPT are a brilliant way to onboard the masses to AI. And it's working. We've come a long way since the 2021 mass hysteria over a DeepFake TikTok of Tom Cruise.
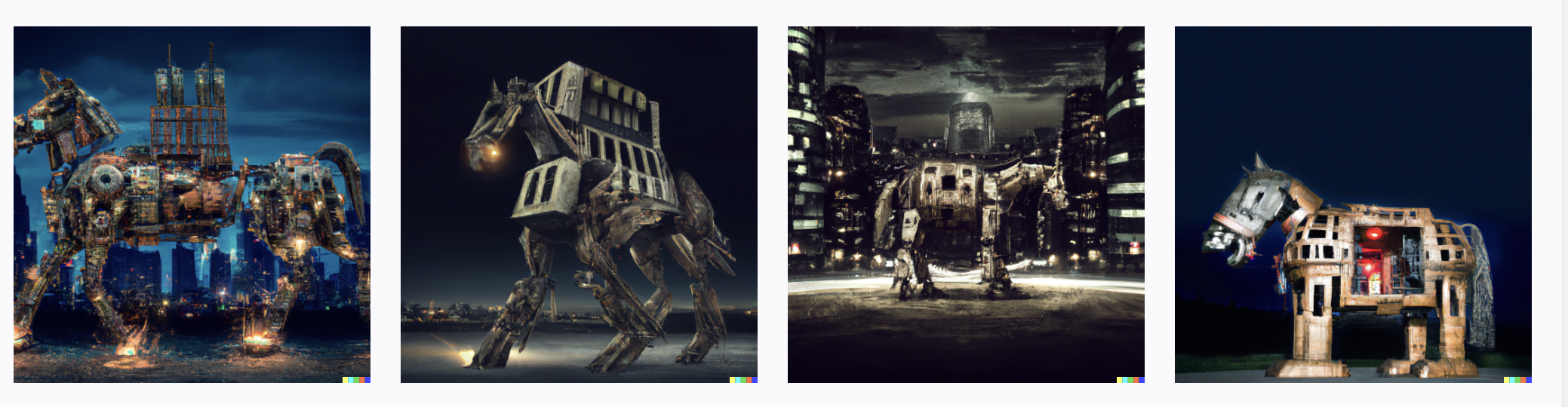
Google is done.
— josh (@jdjkelly) November 30, 2022
Compare the quality of these responses (ChatGPT) pic.twitter.com/VGO7usvlIB
What is Dalle-2
Let's start with Dalle-2 before we get to ChatGPT.
Dalle-2 is an AI model that is able to create images from text descriptions. Dalle-2 went viral over the Summer when its beta was released in July. It was the Summer of generative art. You probably saw pictures on Twitter or Instagram. Basically, everyone used Dalle-2 to turn text into art. Everyone was an amazing artist for a month before the fad wore out.
An example of some "art" I made using Dalle-2. Willing to sell. DM me.
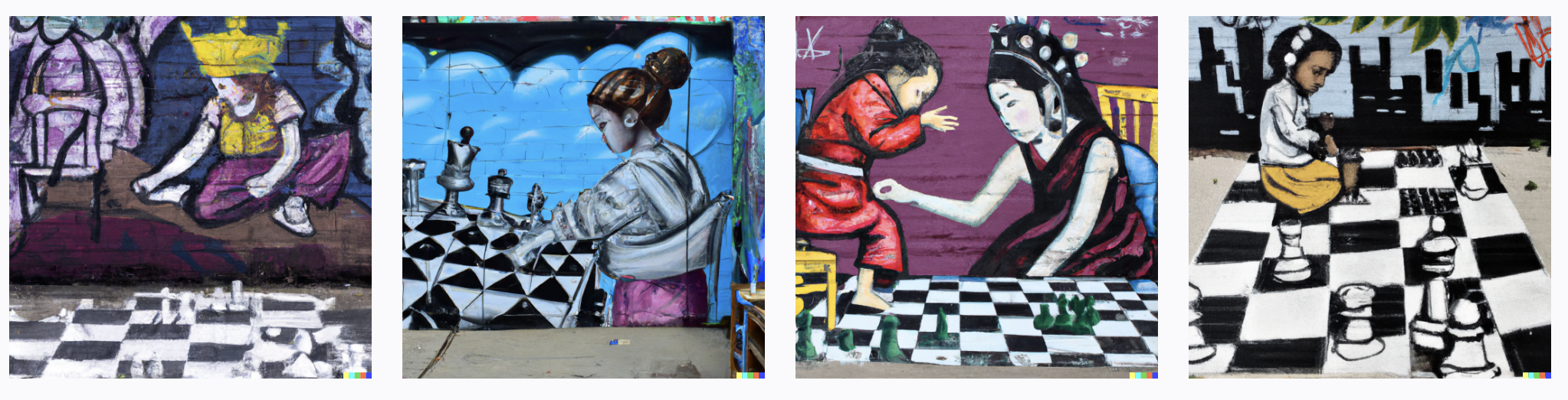
Combining Natural language processing and Computer Vision
Dalle-2 understands the relationships between images and text. It uses a combination of Natural Language Processing (NLP) and Computer Vision (CV) to generate images based on text description. Both NLP and CV are fields that have seen great progress by incorporating AI techniques.
NLP and CV are everywhere. You use the AI daily without even realizing it. NLP is fuel Alexa, Google Search, Gmail, Chatbots, translators etc. On the flip, CV fuels things like Face Unlock, self-driving cars etc.
Dalle-2 basically uses a combination of NLP & CV to:
- Generate images from scratch (text to image).
- Edit or retouch existing images (inpainting).
- Make variants of existing images (variations).
Some more "art" I made using Dalle-2:
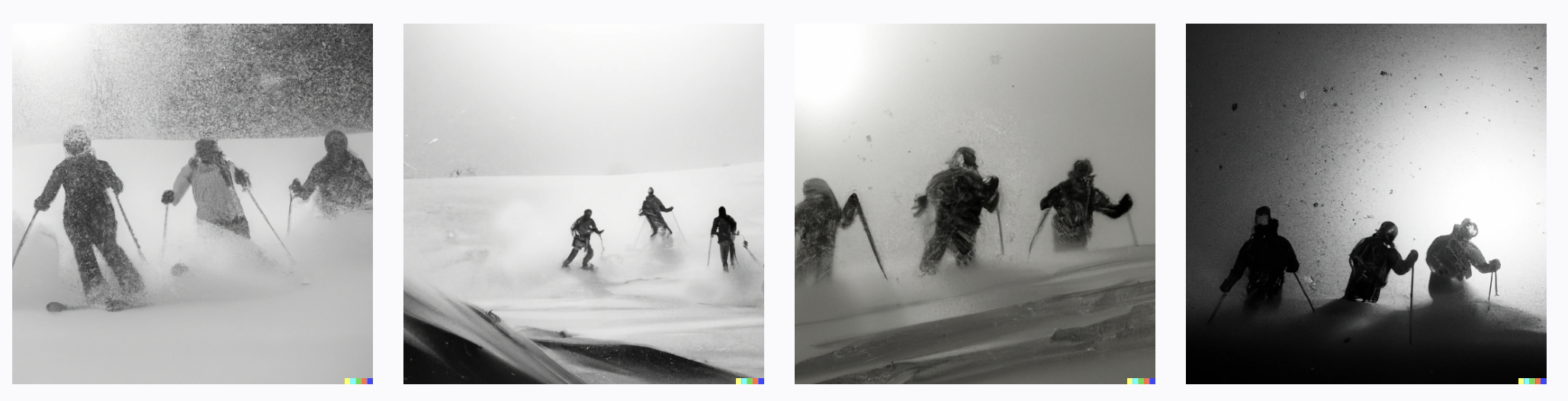
How was Dalle-2 built
My ski art is pretty much made possible by a ton of pictures of people skiing and text describing what's happening in the picture. But what happens then?
Dalle-2 has several stages. Each of them is actually an AI model of its own. The first stage was trained to understand how to link the semantics of text and their visual representations. It’s trained on images and their respective snippets, and learns how relevant snippets are to images. As a by-product, it’s able to produce an embedding, which is a digital signature that allows it to determine how similar or related text snippets and images are.
The second stage was trained to map the text embedding onto a corresponding image embedding. I.e - we now have a way to create a visual representation out of an input text snippet.
The last stage takes the visual embedding and generates images out of it. It effectively churns out variations that preserve the most important characteristics as encoded by the embedding, while altering non-essential details.
AI scientists: let me know if I got anything wrong here.
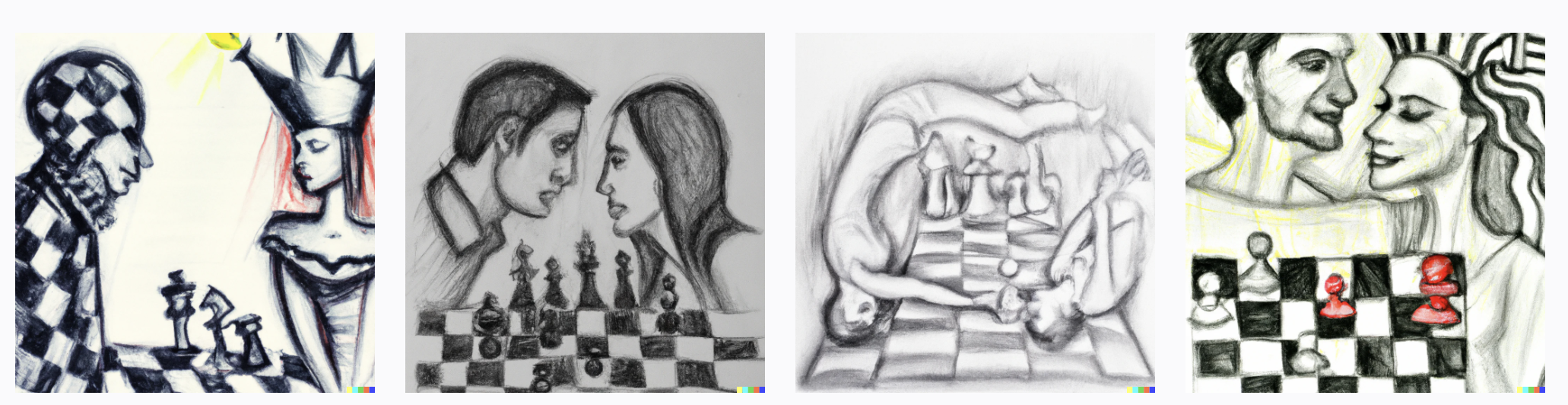
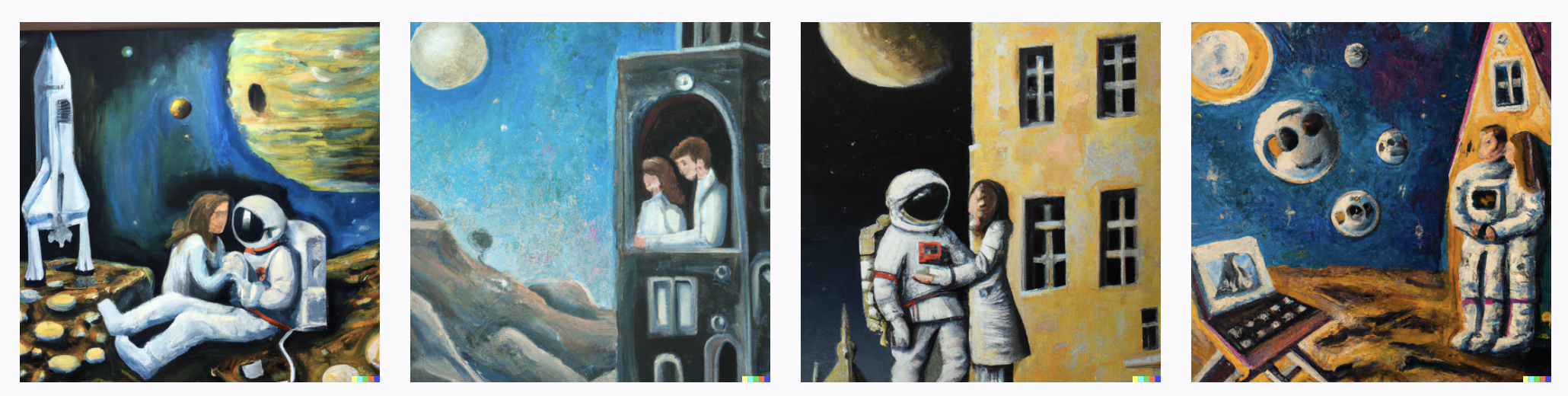
Dalle-2 has limitations
So, I'm not going into depth here because it shouldn't come as a surprise that Dalle-2 has limitations.
- No data: There are data gaps which means that the model performs poorly in some areas.
- Bad data: There are mislabelled images in the dataset. Again, a lot of the data is scraped from the web. Images are going to have the wrong text associated. So the model might throw a result that makes no sense.
I tried to throw some errors. The below is kind of a data error. I guess Dalle-2 doesn't know who SBF is.
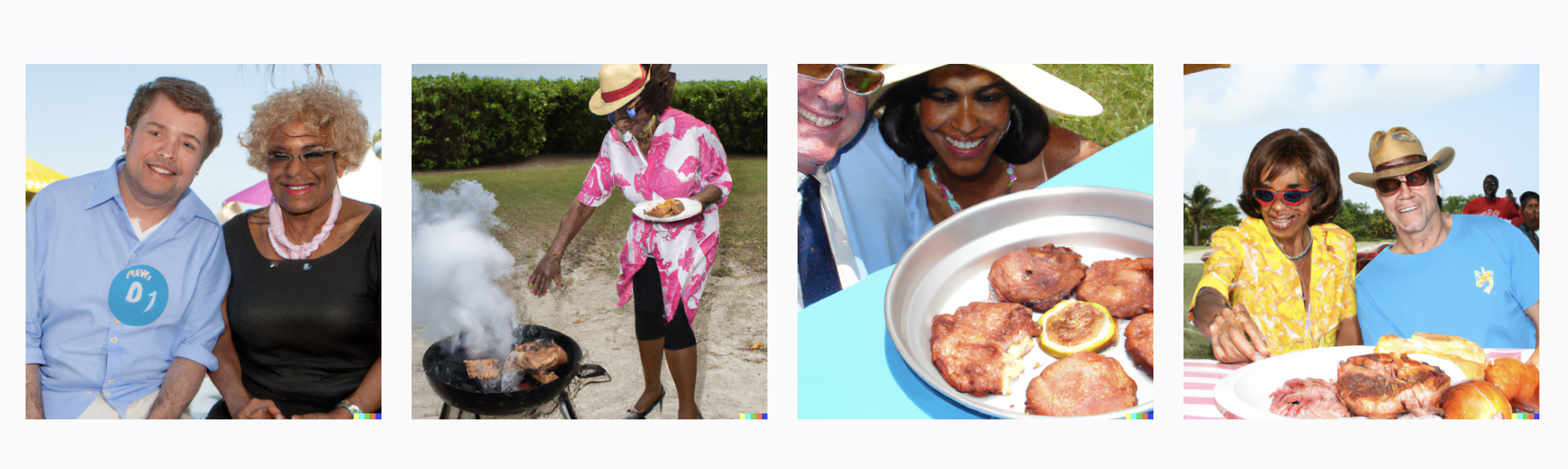
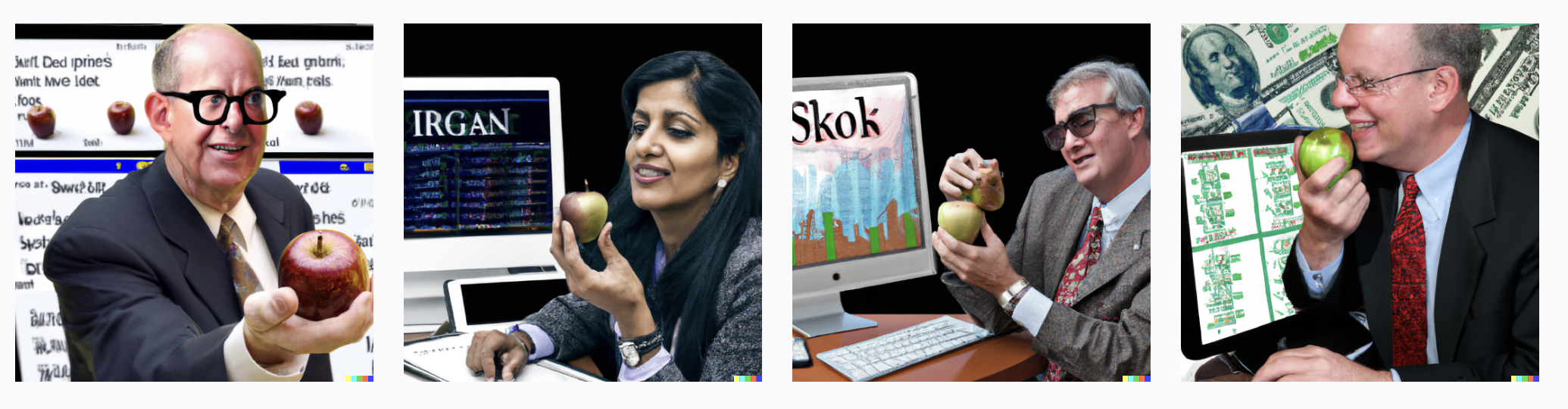
Dalle-2 has risks
Dalle-2 is great. It helps people understand how AI works as well as how people and AI work together. This is a key step forward if we want humans and machines to partner.
There are real risks around Dalle-2. It's all fun and memes on Twitter until it's not. OpenAI put a lot of thought into the risks and put precautions in place. Some examples of types of risks:
- Disinformation: Dalle-2 generates pictures that look real. People could theoretically generate images of fake events and stage them as real.
- Explicit content: ...you get it.
- Bias: Dalle-2 can perpetuate biases by inheriting biases from it's training data. Public datasets are rife with stereotypes. Eg. most images of doctors contain men.
- Botnet: Large scale abuse. I.e. generate a ton of results to spam misinformation on social media or something.
- Reference collisions: Words that reference multiple concepts can lead to malicious collisions. Example of a reference collision? OpenAI uses the eggplant emoji as an example here.
- Data Consent: Users must obtain consent before uploading pictures of others. But how do you practically enforce this? Hard.
OpenAI put precautions in place to mitigate these risks but they aren't comprehensive and difficult to scale. There are policies, human reviewers, and the ability for users to flag content. There are also rate limits that make it harder for "at-scale abuse." The content has also been filtered extensively. I couldn't generate anything politically related or with hot topic names. Sorry Nancy Pelosi.
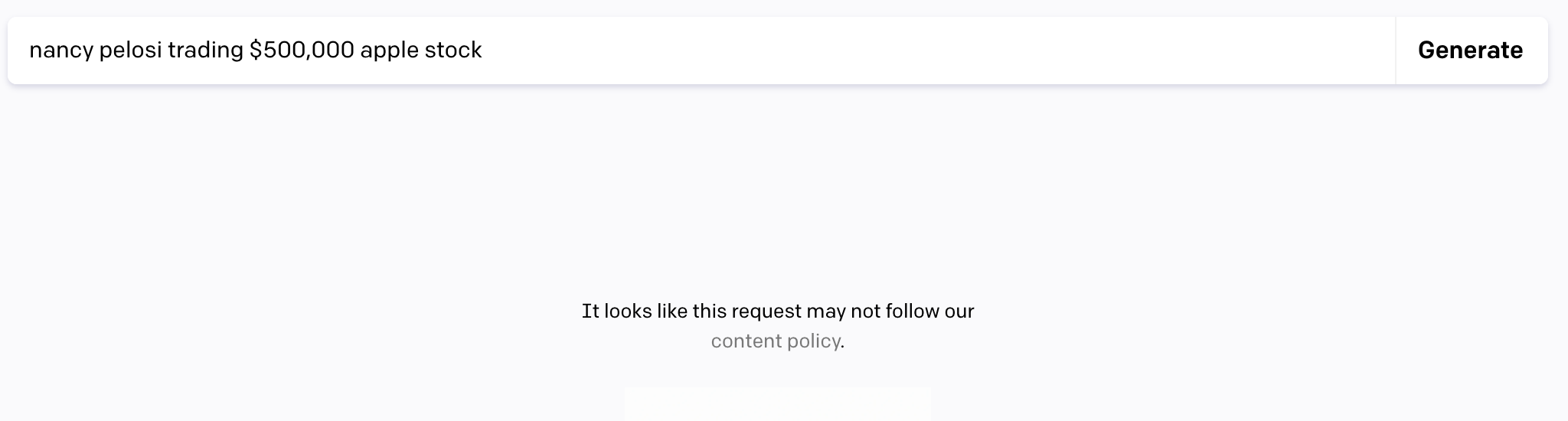
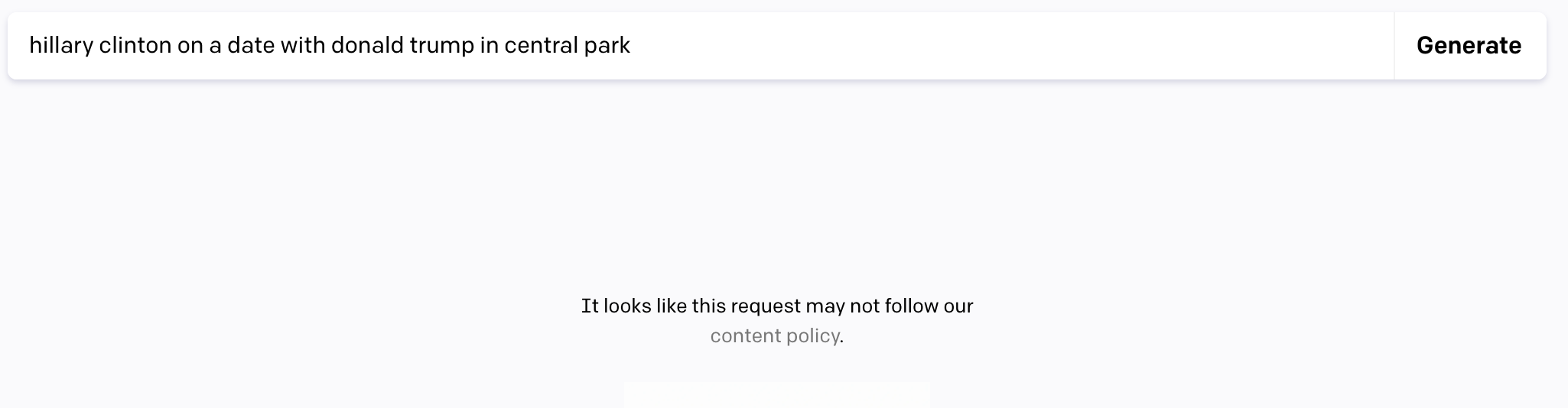
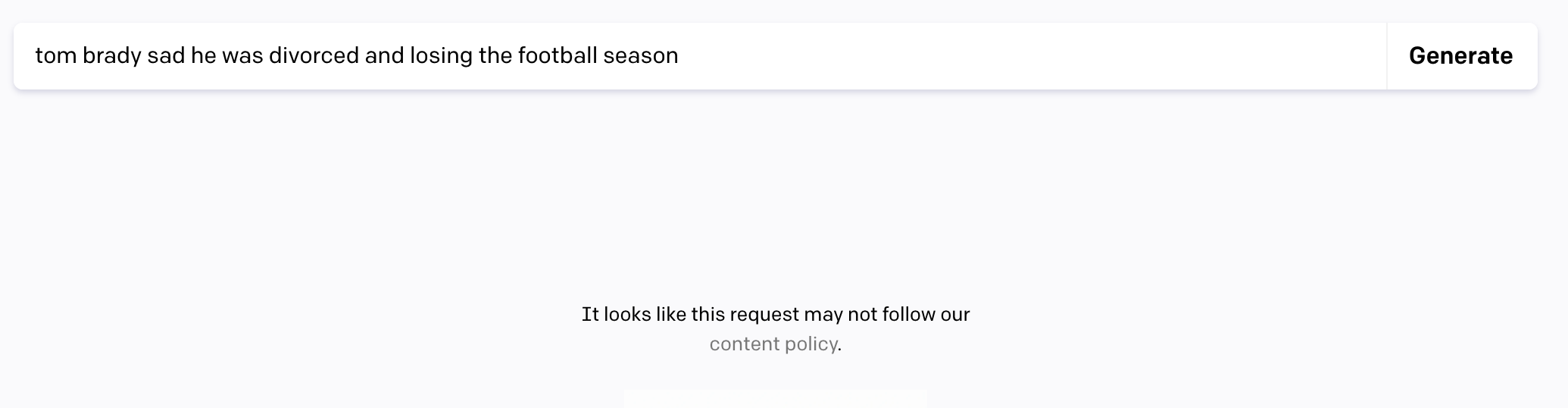
One of the pretty real risks that is hard to get around is the visual synonym problem. OpenAI uses the example of a horse in ketchup to illustrate the visual synonym problem. You can't generate a picture of a horse in blood but you can use visual synonyms like paint or ketchup etc.
People are really clever and have a lot of time on their hands to figure out queries. I'm unsure how OpenAI solves this longer term.
Another real risk? The model at some point will be open sourced. This means that the model could be modified by pretty much anyone.
Dalle-2 what's next?
Dalle has potentially large economic implications around automating certain content related jobs such as photo editing, designing, advertising, modeling etc. Dalle also has a massive opportunity to shape & improve how we create content.
Zooming out, Dalle is part of a broader long pole push on multimodal research at OpenAI. This basically means combining different types of AI to create a more immersive & "worldly" experience.
OpenAI wrote a bit about building out a multimodal experience that is immersive and mimics the real world (well beyond a visual representation.)
So the opposite of:
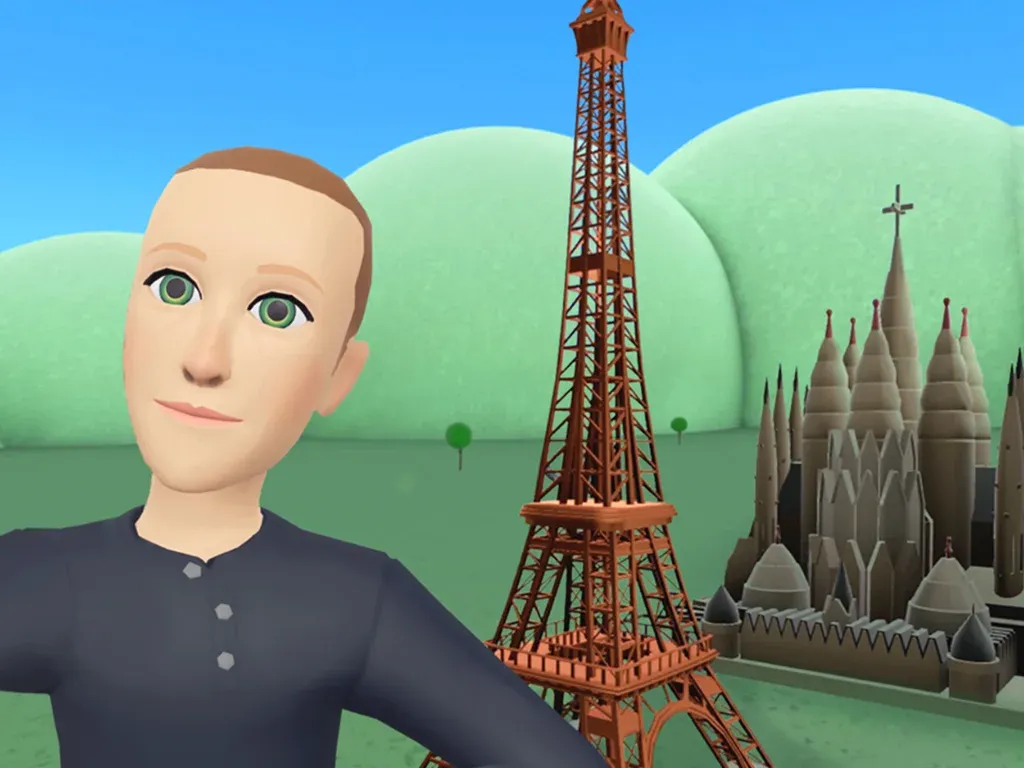
What is ChatGPT
Ok so we went through Dalle-2 in depth. If you made it this far, congrats. On to ChatGPT. Speeding this up...
OpenAI built and released ChatGPT a few weeks ago. Since then, it's pretty much broken the internet and raised real questions around whether it has the possibility to make Google Search obsolete. It's been so popular that there is a waitlist to use it right now. It reached one million users in less than a week.
So what is ChatGPT? ChatGPT is a chatbot on steroids. It has produced pretty mind blowing results and its Q&A functionality really simulates talking to a human. ChatGPT admits to mistakes, challenges questions and lines of thinking etc.
ChatGPT not only simulates conversations around abstract topics but it also can do things like help debug code.
ChatGPT could be a good debugging companion; it not only explains the bug but fixes it and explain the fix 🤯 pic.twitter.com/5x9n66pVqj
— Amjad Masad ⠕ (@amasad) November 30, 2022
How was ChatGPT built
ChatGPT was built off of GPT-3 and fine-tuned to make it really good at conversing with humans. WTF does that mean? Well GPT-3 is a large language model (LLM). Large Language Models (LLMs) can read, summarize and translate texts and predict future words in a sentence letting them generate sentences similar to how humans talk and write. Fine-tuning is the process of taking an existing ML model like an LLM and training it on a dataset specific to a task.
So, ChatGPT basically honed in on the chatbot application of GPT-3 to make it way better and more "real" at conversing with people.
congrats to OpenAI on winning the Turing Test pic.twitter.com/eOuaACKXim
— Max Woolf (@minimaxir) December 6, 2022
GPT-3 had “chatbot” functionality but it had limitations that made it feel less "human", less accurate. For instance, it struggled to synthesize over long trails of questions. It threw errors at repetitions and contradictory statements.
To be clear, GPT-3 is very cutting edge. GPT-3 is one of the most advanced language models out there. It was trained on 45 TB of text data. Data ranges from wikipedia, books, blogs etc. GPT-3 can generate any type of text– it can create poetry, summarize text, generate news articles, stories, and of course answer questions etc.
ChatGPT made strides in solving GPT-3's chatbot limitations in a few ways. To do so, it basically improved the AI by augmenting it with a ton of conversational text. It uses a pretty incredible and super labor intensive dataset.
OpenAI used real people to write conversations where they played both sides of the dialogue. It bootstrapped dialogue by using GPT-3's initial respnse and then making it more robust etc. AI trainers also improved the model by taking results and ranking answers to the same question. I'm sure there were other techniques used to improve the model but I will leave that to the AI scientists.
To summarize how ChatGPT was built in a very simple way...
- Use GPT-3 model to boostrap a model aka use existing AI instead of building from scratch.
- Collect conversational data by generating a question prompt which a human then drafts response to.
- Refine conversation data by generating different answers to the same prompt and then have a human rank results from best to worst.
- Improve model using new data and ranked results.
Again, any feedback from the AI scientists in the room much appreciated.
ChatGPT has limitations & risks
GM. We asked ChatGPT to write us a story about what happened at FTX. pic.twitter.com/f6q6rokmkO
— Autism Capital 🧩 (@AutismCapital) December 2, 2022
As with Dalle-2, ChatGPT has limitations. I should note that the data only runs up to 2021 so more recent topics are a current data gap. Quick overview of limitations...
- Doesn't make sense: ChatGPT can generate answers that sound super smart but actually don't make sense. This is largely because, there is no "right way to answer a conversation." Humans don't make sense half the time.
- Sensitive: Not emotionally. Basically the AI can generate different answers to very similar questions based on small question tweaks.
- Verbose: The model is overly verbose and lengthy in generating text. It can also repeat phrases or hot words a ton. Both issues are due to biases in the training data. A lot of the human written responses were too long (like this blog). Certain words or answers were more frequent in the data.
- Imprecise: The model more often than not guesses user intention rather than "asking for clarification." But again this is super common in human conversations too.
I personally don't see these as big issues. Half of the time when I talk to friends, I don't understand what they are talking about.
But like Dalle-2, ChatGPT can use user generated content to improve the model and augment the underlying datasets. Doing so will only improve precision and further humanize the AI.
ChatGPT risks are pretty similar to Dalle-2's so I won't belabor. TLDR; it can perpetuate biases, it can potentially generate harmful content, it can facilitate spread of misinformation or disinformation.
OpenAI put risk mitigations in place and it largely rejects harmful prompts. But humans are crafty and it is easy to get around.OpenAI is highly encouraging users to provide input on response quality. There is even a ChatGPT Feedback Contest (not a large prize, don't get excited.) Main takeaway? Deployment is an iterative process...
ok I think I broke it pic.twitter.com/nXR87PNrhc
— vitalik.eth (@VitalikButerin) December 1, 2022
ChatGPT what's next?
ChatGPT is scary good. We are not far from dangerously strong AI.
— Elon Musk (@elonmusk) December 3, 2022
I personally think that ChatGPT has the opportunity to overtake Google Search.
Google's mission is to "organize the world's information." That's great but the shift from organizing to understanding and explaining the world's information is way more powerful.
The ability for machines to converse and discover with humans or for humans is a powerful accelerant. Humans and machines can do more, together.
A quote with Sam Altman who cofounded OpenAI to close this blog out:
“Soon you will be able to have helpful assistants that...give advice...goes off and does tasks for you...discovers new knowledge for you.”
Conclusion
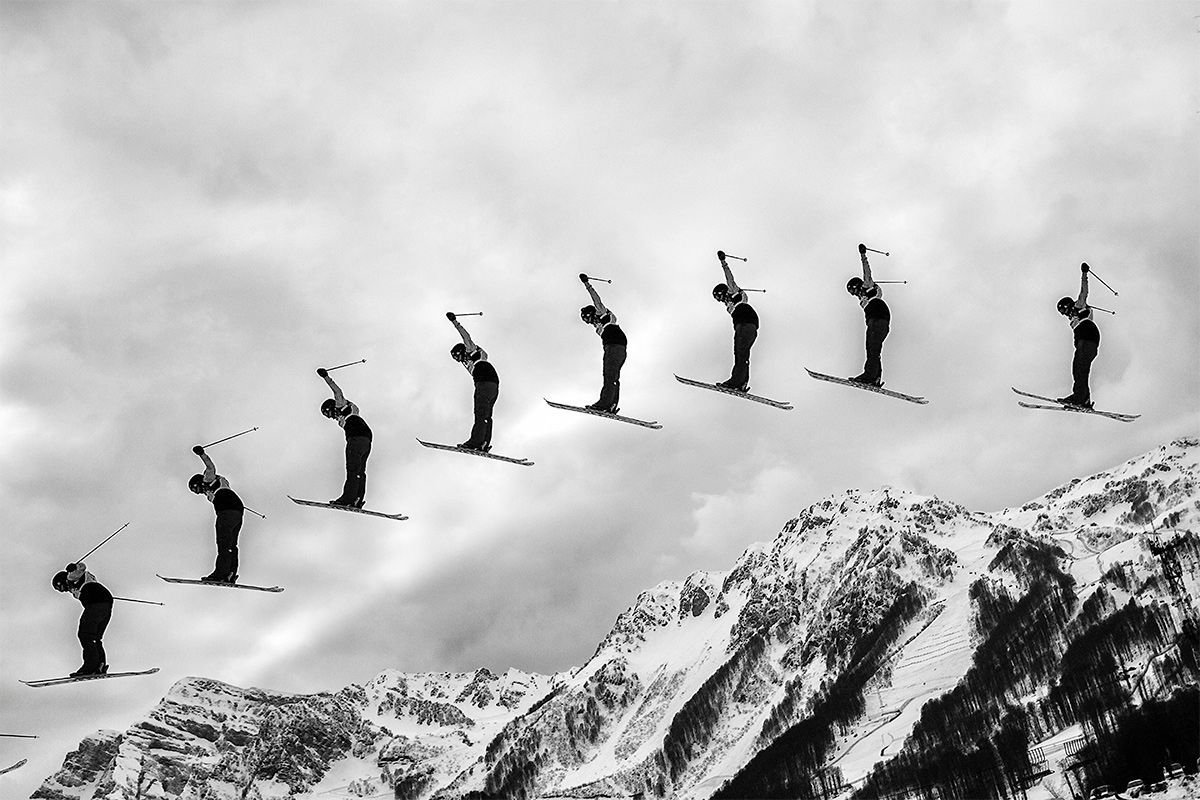
Onboarding to AI is here. ChatGPT and Dalle-2 have taken AI mainstream. Yes, the masses use AI every day but most are just not aware of the extent. Most don't even realize that auto-complete is a form of AI.
Being aware of AI, excited by it, and comfortable with it...is a massive step forward. I guess the next step is to see just how comfortable we can get normal people with AI. And then understand which forms of AI help humans do more, learn more, achieve more.
Thanks for reading. Feedback? Drop a comment or DM.
Shout out to Tomer Meron, my former boss and mentor for proofing.
This blog was generated by ChatGPT.
(JK, I wrote it).